May 2024
Policy Brief: Understanding and Improving Early Intervention Systems
Projects
This policy brief is a summary of a research paper entitled “Predicting Police Misconduct” by Greg Stoddard, Dylan Fitzpatrick, and Jens Ludwig.
Most of the public discussion about police misconduct in America has focused on what to do after a tragedy occurs – should the officer be disciplined or even prosecuted, should they be allowed to move to a new department and continue working as a police officer, and how can we put into place trustworthy systems for investigating police misconduct, etc. Those are important questions, but in some sense, they are too late. Ideally, we would like to identify a way to prevent misconduct from occurring in the first place, which would spare members of the public from experiencing harm – and help save the careers of officers themselves.
One strategy to prevent misconduct is to use an early intervention system (EIS), which is meant to identify officers that show warning signs of risk for an adverse event. The hope is that this early identification allows a police department to intervene with supports, services, or training before the next event occurs.
But any EIS will only be helpful if it is able to effectively predict misconduct – that is, to identify those officers at highest risk for future misconduct or other harmful outcomes. Unfortunately, many of these systems to date are not actually predictive. Despite the statistical goal of these systems, their design – including what data elements should count as a risk factor and how to weigh those factors – is often dictated by some combination of what is effectively guesswork and/or legal negotiations, rather than data analysis.
In 2016, the University of Chicago Crime Lab partnered with the Chicago Police Department (CPD) to research and build an early intervention system that would truly be data driven. This brief summarizes the key lessons from our extensive research on over a decade of CPD data. Those lessons include:
- A data-driven system can identify officers at significantly elevated risk for misconduct, but the level of accuracy is far from perfect. While predictive models can help prevent misconduct, no EIS will be a panacea.
- Predicted risk of misconduct is not simply a proxy for policing activity. Officers with very similar levels of measured activity (arrests, guns confiscated, etc.) can vary enormously in their risk of future misconduct.
- Risk of on-duty and off-duty misconduct are correlated. This suggests that officer wellness interventions may help reduce both on-duty and off-duty adverse events.
- While EI systems often focus on ‘serious events’ as warning signs, our data analysis suggests what matters more is an officer’s larger pattern of events. For example, an officer’s entire record of complaints is significantly more predictive than just their record of prior sustained complaints.
- Police departments can get most of the benefits of a fully-blown predictive model at much lower cost with a simple policy based on count of prior complaints from the past two years.
For a longer discussion of these issues, please see our research paper.
Background
In 2016, the University of Chicago Crime Lab partnered with the Chicago Police Department to research and build an early intervention system that would truly be data-driven. Our approach to this problem differs from most prior systems in two important ways.
First, we were explicit about the type of negative outcomes that the system is designed to predict. This project focused on two types of negative outcomes – on-duty misconduct, which are defined as sustained allegations of excessive force, wrongful arrest, or improper stops, and off-duty misconduct which include any complaint related to domestic violence, drug or alcohol use, or other off-duty activity.
Second, once we selected those outcomes as the target of the prediction, we combined a large historical database of CPD records from 2008-2020 with the tools of machine learning to identify the factors that were the most predictive of these outcomes. The result of this analysis is two risk models – one that predicts risk of on-duty misconduct based on an officer’s records from the prior five years, and one that predicts risk of off-duty misconduct based on records from the prior five years. This policy brief outlines the primary findings from this research.
Misconduct is (somewhat) predictable
We find that data-driven systems can forecast misconduct risk but the methods are not perfect. The top 2% of officers by predicted on-duty risk account for 10% of all on-duty misconduct – or put differently, compared to the average officers, those with the highest predicted risk are 5 times more likely to be involved in an on-duty misconduct. We similarly find that the top 2% of officers by predicted off-duty risk account for 10% of all off-duty misconduct. On the other hand, it is important to acknowledge that there are real limits to predictability of misconduct. Most misconduct – both on-duty and off-duty – involve officers who would not have been identified as high risk.
These results show that the right way to think about an EIS is not as a panacea that will solve the problem of misconduct, but as one tool to help direct attention and resources where they will have an outsized impact. If a department can give a helpful or preventative resource to all officers, they absolutely should. However, the reality is that effective supports are likely too resource intensive to give to every officer. Our findings suggest there are real benefits from using data to target those supports.
Insights into the nature of misconduct risk
Our research also reveals several new insights about the underlying nature of risk. The first insight is that risk of misconduct is not simply a proxy for policing activity (arrests, stops, etc). One frequent concern with an EIS is that it will conflate risk with activity, and in turn, will flag the most active officers rather than the riskiest. We tested that concern directly and did not find support for it. Less than half of the differences in risk scores between officers can be explained by differences in activity like number of arrests or guns recovered. Put differently, officers with similar assignments and activity can differ enormously in predicted risk of misconduct.
The second insight is that many officers identified as high-risk for on-duty misconduct are also at elevated risk for off-duty misconduct, suggesting that reducing misconduct and increasing officer wellness may be two sides of the same coin. Given that so much of the policy conversations centers around on-the-job misconduct, many of the suggested interventions are things like additional training or body-camera reviews. However, our data analysis (and conversations with front-line police officers) suggests that efforts to prevent on-the-job misconduct could benefit from adequate supports for officers to deal with both on-duty and off-duty challenges.
Implications for designing EIS and other systems
Finally, our research has implications for the design of EIS and other systems – such as misconduct databases – beyond Chicago.
Our analysis reveals that the most important risk factor is a pattern of prior complaints, and that the specific details of prior complaints (such as the specific category or the investigatory outcome of those complaints) carry little additional predictive signals. We summarize this insight as “focus on patterns, not events” as a good mental model for thinking about risk. Building on that insight, we find that a simple policy that flags officers with the most prior complaints in the past two years is accurate enough to help target resources and interventions.
This result has several policy implications.
First, it suggests that benefits of a data-driven prediction and targeting of supports is more practically available than it may seem. While a machine learning model is the most accurate way to predict risk, they also require a large dataset and significant resources to implement. Our results suggest that a second-best approach – ranking by prior complaints – may achieve most of the benefits from the machine learning model without those same costs. This is particularly relevant for smaller, which will likely lack the sample size and budget to develop such an EIS, but account for ~63% of police killings.
A different implication or question raised by our finding of “focus on patterns, not events” is whether to restrict attention to just sustained complaints or to build data systems that capture and draw on data from all complaints. Our results show that all complaints – even complaints that are not sustained or are still pending – have predictive signal. In fact, restricting the risk models to just sustained complaints degrades the accuracy of the risk models to the point where the risk flags are not much better than random guessing. However, using all prior records of misconduct can introduce procedural concerns and the possibility of affecting an officer’s career based on unfounded allegations. Our findings suggest this is one of the most important (if not the most important) tradeoff that policymakers face when designing risk management systems and databases.
A key priority for future research is to better understand what types of preventative interventions are most useful in practice. While our work sheds light on how departments might use their data to better target interventions, these systems will only be as good as the interventions themselves.
Projects
Latest Updates
U. of C. study shows cops at high risk of misconduct also at elevated risk for off-duty trouble
The Chicago Tribune’s Caroline Kubzansky speaks with Crime Lab Senior Research Director Greg Stoddard to discuss results from a new study of an officer support system.
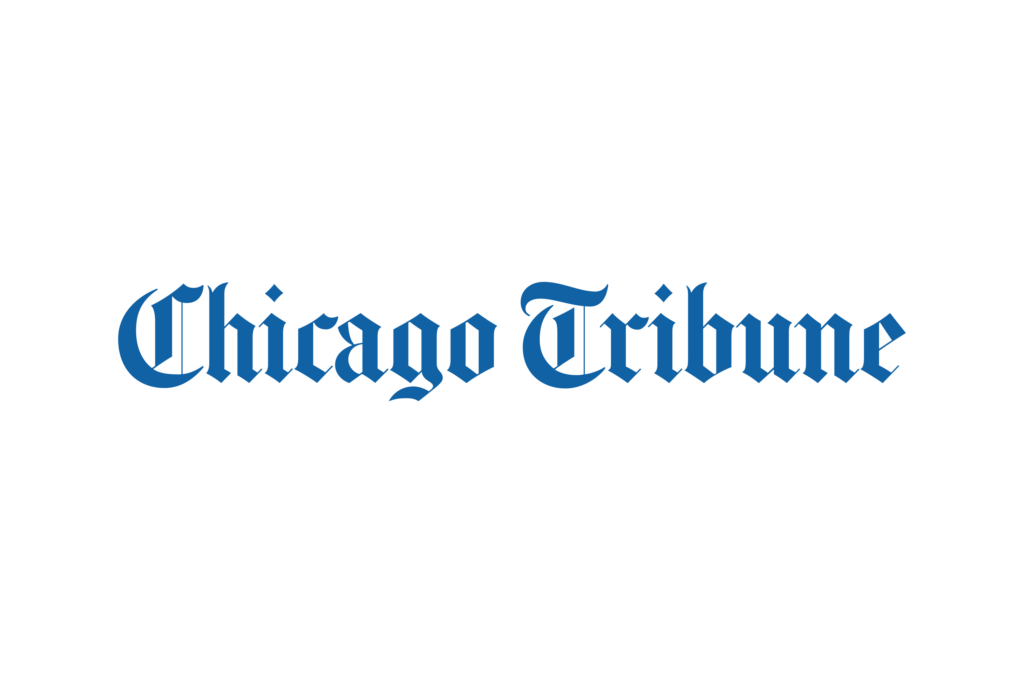
Reset with Sasha-Ann Simons: Can police misconduct be stopped before it starts?
Crime Lab Senior Research Director Greg Stoddard joins Patrick Smith on WBEZ Reset to discuss results from a new study of an algorithm that can help identify which officers are likely to commit misconduct.
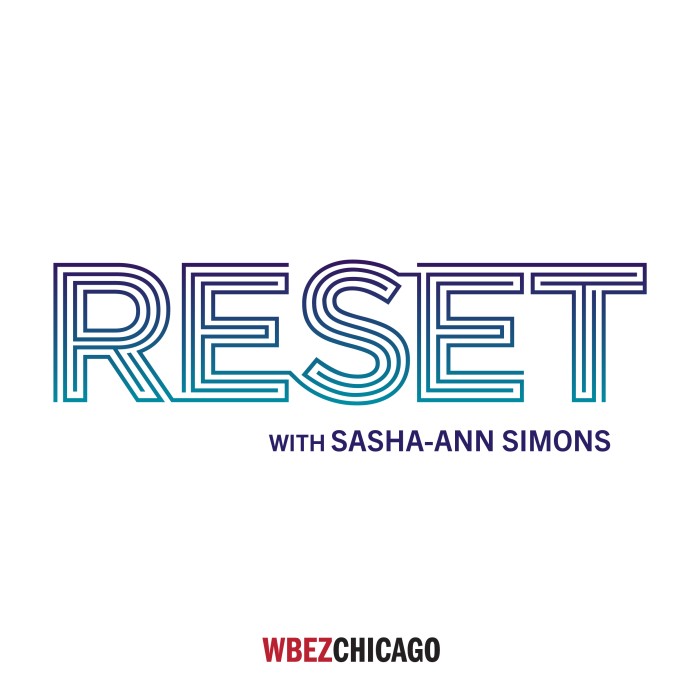
Situational Decision-Making: A New Training to Improve Policing
We invite you to join us for a webinar on the findings of our recently released study, A Cognitive View of Policing.
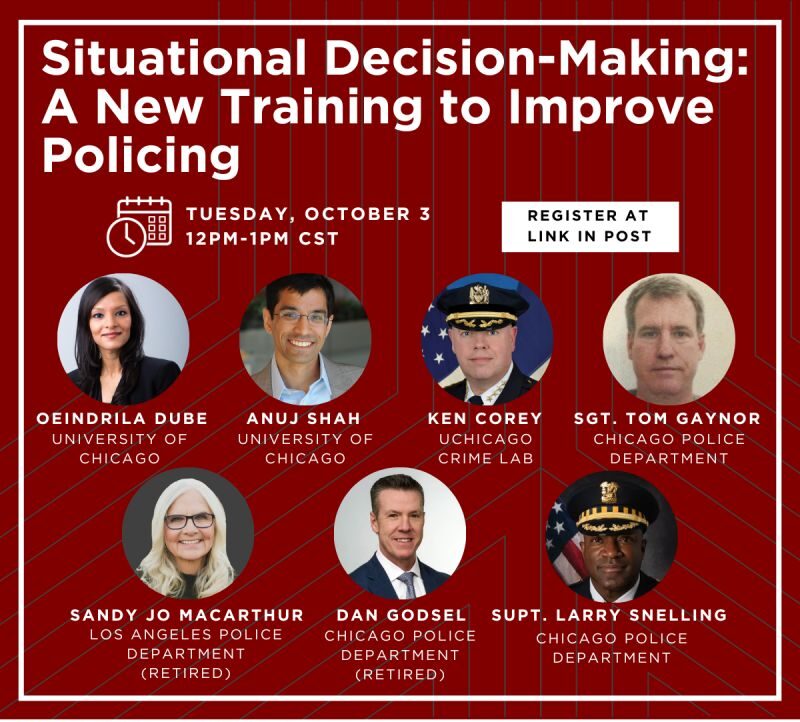