Officer Support System (OSS)
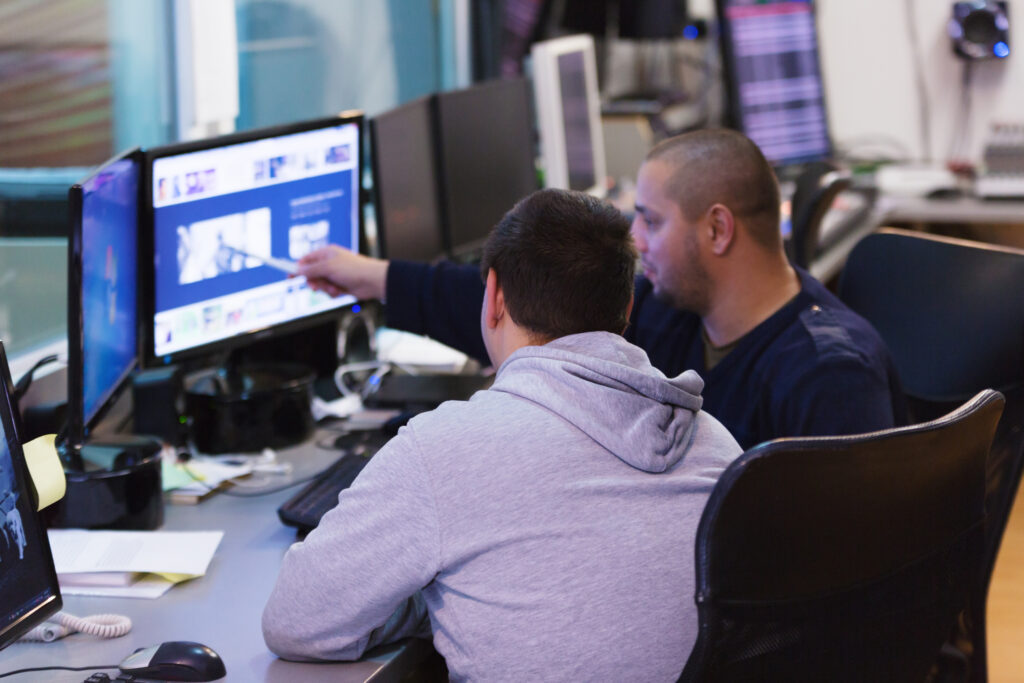
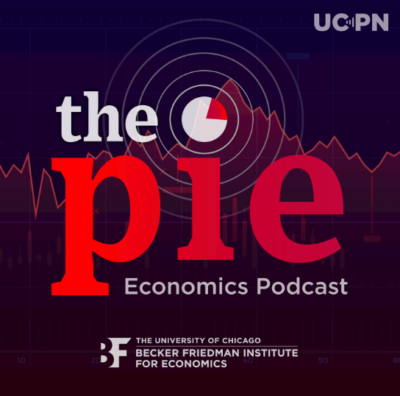
Using Machine Learning to Predict—and Prevent—Police Misconduct
In this episode of The Pie, Greg Stoddard, Senior Research Director for the Crime Lab and Education Lab, discusses recent research using administrative data from the Chicago Police Department to predict officers misconduct, before it happens.
The Crime Lab partnered with the Chicago Police Department (CPD) to develop the Officer Support System (OSS), a next-generation, data-driven early intervention system that aims to identify patterns of officer behavior predictive of a future adverse outcome and intervene to provide support.
Challenge
We have seen a decline in public trust in the police and a simultaneous, and surely not coincidental, decline in morale among front-line police officers. To reduce harmful impacts on residents, improve police-community trust, boost morale among front-line officers, and improve officer wellness more generally, we must figure out how to prevent these tragedies from happening in the first place.
Opportunity
The OSS aims to identify patterns of officer behavior predictive of future misconduct, find officers currently displaying those behaviors, and intervene to provide support. The project, which has been underway since 2016, offers an opportunity for Chicago to develop one of the country’s first data-driven early intervention systems.
Solution
The study shows it is possible to predict risk of on-duty and off-duty misconduct. Using estimates from a data-driven algorithm that predicts an officer’s future risk of serious misconduct from their past record of activity and complaints against them, we find that officers in the top 2% of the predicted risk distribution are 6 times more likely to engage in serious misconduct than the average officer. While this level of predictability is certainly far from perfect, it allows the Chicago Police Department to target supervisor time and supportive resources like training and mental health services to those who will benefit most.
Project overview
Most of the public discussion about police misconduct in America has focused on what to do after a tragedy occurs: Should the officer be disciplined or even prosecuted? Should they be allowed to move to a new department and continue working as a police officer? How can we put into place trustworthy systems for investigating police misconduct?
These are important questions, but what if we could identify a way to prevent misconduct from occurring in the first place? Police departments already have a number of support systems in place, but these supports are costly, and local government budgets are tight. That means we can’t give support interventions to every officer on the force; we need to prioritize and target these resources to officers who need help the most, and in the best of cases, prevent misconduct from occurring in the first place.
One strategy to prevent misconduct is to use an early intervention system (EIS) to identify officers that show warning signs of risk for an adverse event. Successful early identification allows a police department to intervene with supports, services, or training before an adverse event occurs.
But an EIS is only helpful to the extent that it can effectively predict misconduct – that is, to identify those officers at highest risk for future misconduct or other harmful outcomes. Unfortunately, many of these systems are not actually predictive in practice. Despite the statistical goal of these systems, their design – including what data elements are considered risk factors and how to weight those factors – is often dictated by some combination of guesswork, intuition, and/or legal negotiations, rather than rigorous data analysis.
Years Active
2016 – present
Topics
Methodologies
Project Leads
Dylan Fitzpatrick
Research Director
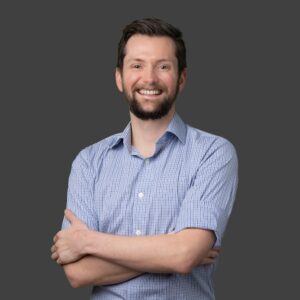
Maggie Goodrich
CEO, TacLogix, Inc.
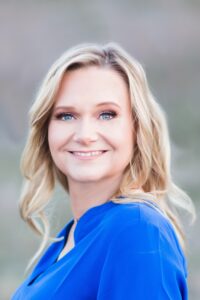
Katie Larsen
Research Manager
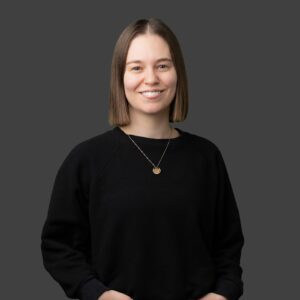
Jens Ludwig
Pritzker Director
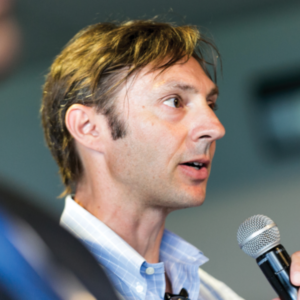
Greg Stoddard
Senior Research Director
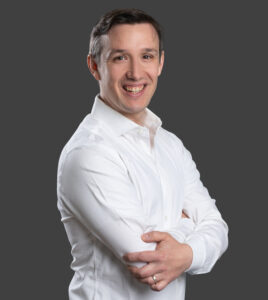
In 2016, the University of Chicago Crime Lab partnered with the Chicago Police Department (CPD) to build an early intervention system based on a statistical analysis of 10+ years of CPD data. Our goal was to create a more accurate EIS by using statistics and machine learning to discover the most predictive risk factors, and to understand the extent to which risk of misconduct could be predicted in the first place.
Using estimates from a data-driven algorithm that predicts an officer’s future risk of serious misconduct from their past record of activity and complaints against them, we find that the top 2% of officers with highest predicted risk are 6 times more likely to engage in serious misconduct than the average officer. While this level of predictability is far from perfect, it provides an enormously helpful decision aid for targeting supportive resources, enabling the Chicago Police Department to direct supervisor time and training and mental health services to those who will benefit most.
- Our work revealed several key lessons relevant both to the Chicago Police Department and other departments around the country. A data-driven system can identify officers at significantly elevated risk for misconduct, but the level of accuracy is far from perfect. While predictive models can help prevent misconduct, no EIS will be a panacea.
- Predicted risk of misconduct is not simply a proxy for policing activity. Officers with very similar levels of measured activity (arrests, guns confiscated, etc.) can vary enormously in their risk of future misconduct.
- Risk of on-duty and off-duty misconduct are correlated. This suggests that officer wellness interventions may help reduce both on-duty and off-duty adverse events.
- While EI systems often focus on ‘serious events’ as warning signs, our data analysis suggests what matters more is an officer’s larger pattern of events. For example, an officer’s entire record of complaints is significantly more predictive than just their record of prior sustained complaints.
- Police departments can get most of the benefits of a fully-blown predictive model at much lower cost with a simple policy based on count of prior complaints from the past two years.
Latest Updates
U. of C. study shows cops at high risk of misconduct also at elevated risk for off-duty trouble
The Chicago Tribune’s Caroline Kubzansky speaks with Crime Lab Senior Research Director Greg Stoddard to discuss results from a new study of an officer support system.
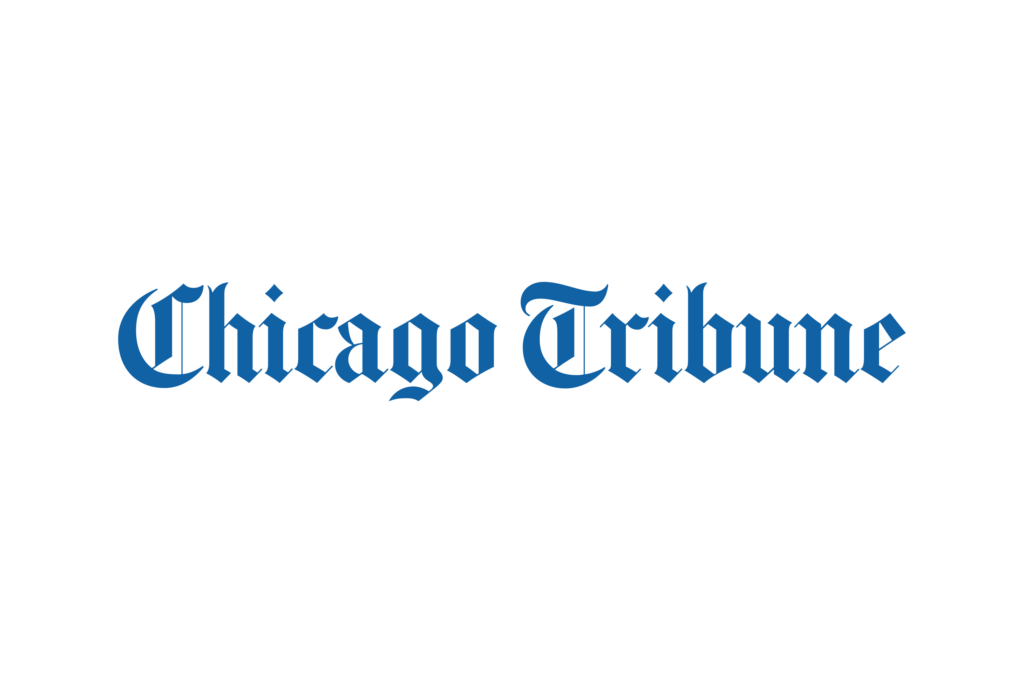
Reset with Sasha-Ann Simons: Can police misconduct be stopped before it starts?
Crime Lab Senior Research Director Greg Stoddard joins Patrick Smith on WBEZ Reset to discuss results from a new study of an algorithm that can help identify which officers are likely to commit misconduct.
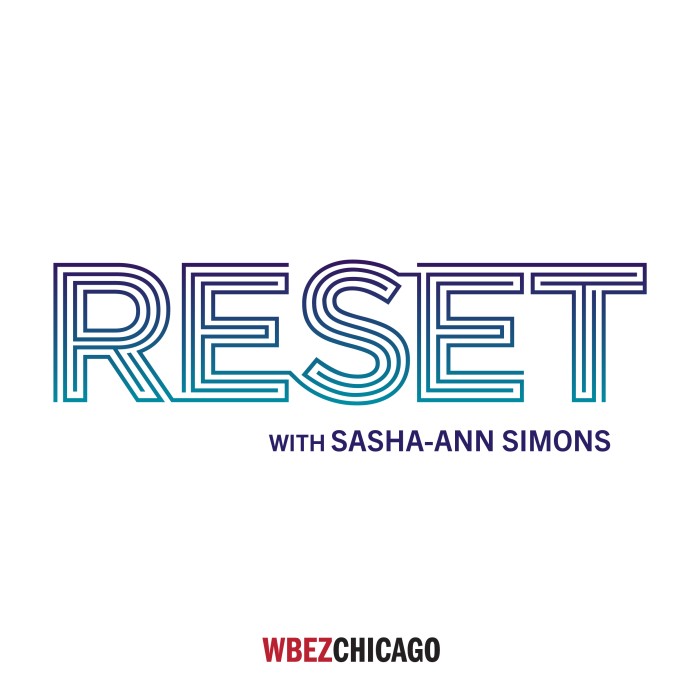
UChicago Crime Lab Study Finds Officer Support Systems Can Use Data to Predict Risk of Police Officer Misconduct, Offers a Low-Cost Decision Aid for Targeting Resources
Findings from a study of an officer support system using Chicago Police Department data show that it is possible to predict an officer’s future risk of serious misconduct.
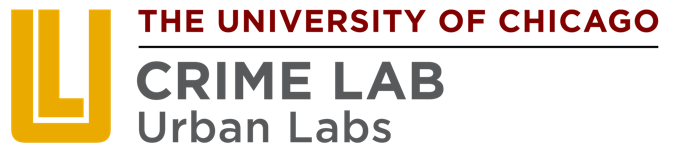
NBER Working Paper: Predicting Police Misconduct
This paper outlines the results of research on over a decade of Chicago Police Department data that shows it is possible to predict risk of on-duty and off-duty misconduct, allowing police departments to prioritize training and supportive resources.
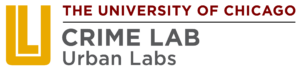
Policy Brief: Understanding and Improving Early Intervention Systems
This policy brief is a summary of a research paper entitled “Predicting Police Misconduct” by Greg Stoddard, Dylan Fitzpatrick, and Jens Ludwig.
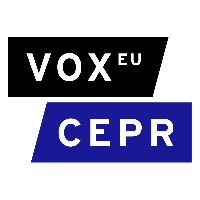
Getting More Out of Policing in the US
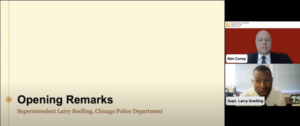
Webinar – Situational Decision-Making: A New Training to Improve Policing
The Crime Lab hosted a webinar on the findings of our recently released study, A Cognitive View of Policing, which evaluated a pilot of the Situational Decision-Making (Sit-D) police training program.