UChicago Crime Lab Study Finds Officer Support Systems Can Use Data to Predict Risk of Police Officer Misconduct, Offers a Low-Cost Decision Aid for Targeting Resources
Findings from a study of an officer support system using Chicago Police Department data show that it is possible to predict an officer’s future risk of serious misconduct.
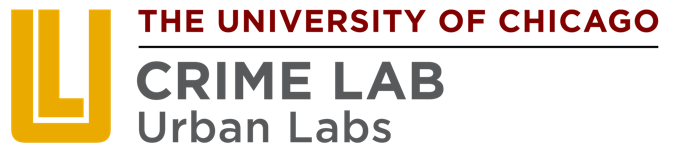
CHICAGO, IL – The University of Chicago Crime Lab released results of research on over a decade of Chicago Police Department data that shows it is possible to predict risk of on-duty and off-duty misconduct. The paper, titled Predicting Police Misconduct, was published by the National Bureau of Economic Research. The research uses estimates from a data-driven algorithm that predicts an officer’s future risk of serious misconduct from their past record of activity and complaints against them. The results show that the top 2% of officers with the highest predicted risk are 6 times more likely to engage in serious misconduct than the average officer. While this level of predictability is far from perfect, it provides a helpful decision aid for targeting supportive resources, enabling the Chicago Police Department to direct supervisor time and training and mental health services to those who will benefit most.
“Police misconduct is enormously harmful to the public, to the careers of officers, and trust between police departments and the communities they serve,” said Dr. Greg Stoddard, Senior Research Director at the University of Chicago Crime Lab. “This research shows that it’s possible to use data to identify where supports and services would do the most good. More importantly, our work shows that simple policies can be effective tools at very low costs.”
This project began in 2016 when the University of Chicago Crime Lab partnered with the Chicago Police Department (CPD) to build an early intervention system based on a statistical analysis of 10+ years of CPD data. The goal of the partnership was to create a more accurate early intervention system by using statistics and machine learning to discover the most predictive risk factors, and to understand the extent to which risk of misconduct could be predicted in the first place.
Our work revealed several key lessons relevant both to the Chicago Police Department and other departments around the country.
- A data-driven system can identify officers at significantly elevated risk for misconduct, but the level of accuracy is far from perfect. While predictive models can help prevent misconduct, no EIS will be a panacea.
- Predicted risk of misconduct is not simply a proxy for policing activity. Officers with very similar levels of measured activity (arrests, guns confiscated, etc.) can vary enormously in their risk of future misconduct.
- Risk of on-duty and off-duty misconduct are correlated. This suggests that officer wellness interventions may help reduce both on-duty and off-duty adverse events.
- While EI systems often focus on ‘serious events’ as warning signs, our data analysis suggests what matters more is an officer’s larger pattern of events. For example, an officer’s entire record of complaints is significantly more predictive than just their record of prior sustained complaints.
- Police departments can get most of the benefits of a fully-blown predictive model at much lower cost with a simple policy based on count of prior complaints from the past two years.
This project was supported by the Irving Harris Foundation, Griffin Catalyst, the Joyce Foundation, the Charles Koch Foundation, the MacArthur Foundation, the Motorola Solutions Foundation and the U.S. Department Of Justice’s Bureau of Justice Assistance.
###