New York City Domestic Violence Analysis
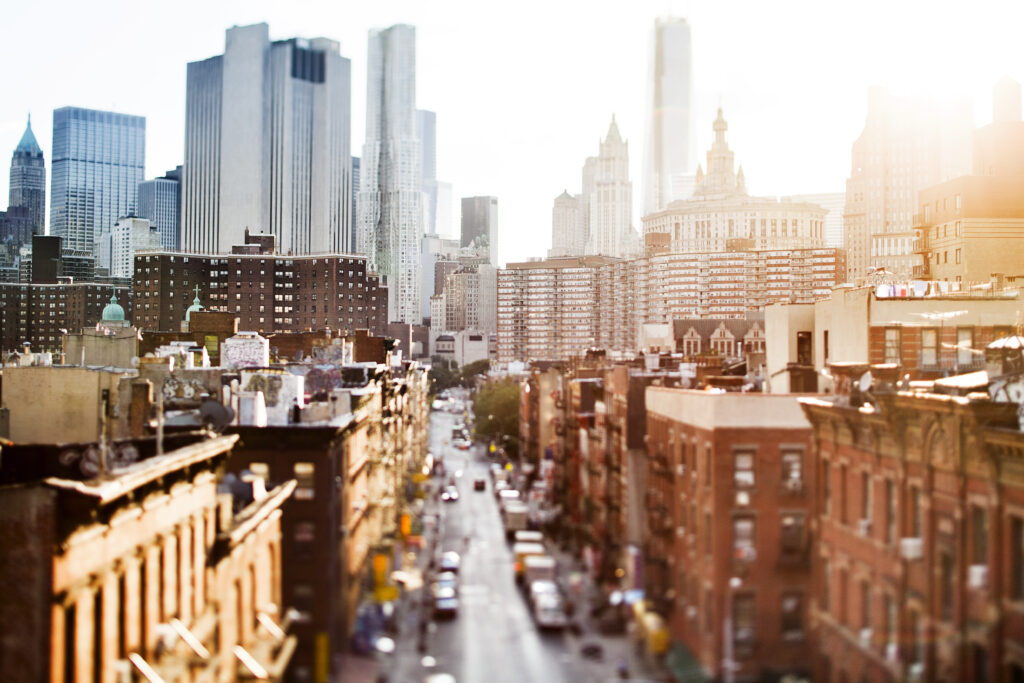
The Crime Lab partnered with the New York City Police Department (NYPD) to identify New Yorkers at high risk of domestic violence to ensure those individuals received social services.
Challenge
Domestic violence impacts thousands of New Yorkers each year, but chronic underreporting makes it difficult for public agencies to develop targeted outreach efforts and efficiently offer services for survivors.
Opportunity
To combat this challenge and improve support service delivery, the Crime Lab used historical crime data to develop a machine learning model to identify individuals at high risk of domestic violence re-victimization. The model’s performance suggests that these tools can be useful for targeting domestic violence interventions and can be built using data that most police departments already collect.
Project overview
Over the past 25 years, even as New York City’s overall homicide rate declined by 85%, the share of domestic violence-related homicides more than tripled. In 2016, the Crime Lab partnered with the New York Police Department (NYPD) to develop a machine-learning model that predicts which domestic violence survivors are most likely to experience another domestic violence incident. The prediction model relies on machine learning to process over ten years of NYPD’s domestic violence incident data, including statements made by survivors and responding officers. These predictions were used to prioritize the NYPD’s outreach and response efforts and to improve the allocation of the city’s limited resources for domestic violence survivors. The model was successful in accurately identifying individuals at high risk of re-victimization, reinforcing the importance of machine learning as a tool for public service delivery and for answering research questions about the data needed to predict domestic violence incidents.
Years Active
2015
Topics
Project Leads
Melissa McNeill
Data Science Manager
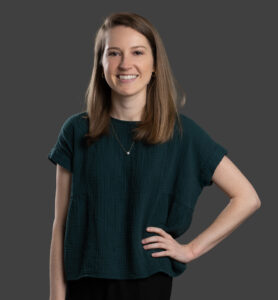
Zubin Jelveh
Assistant Professor, College of Information Studies at the University of Maryland
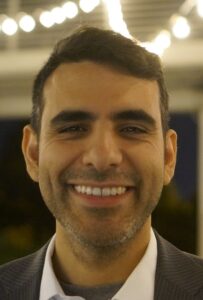